Waypoints to the Summit: Tracing AI's Forecasting Parallels
Charting the journey from fantasy to precise predictions in weather forecasting and AI, this article shows how innovations build on each other, turning impossible dreams into everyday tools.
Innovation often progresses one step at a time, each milestone building on those before. Weather forecasting epitomizes this climb, its history transforming fantasy into precise science. For centuries, weather predictions relied on lore passed down through generations. It was not until the 20th century that the needed mathematical models were discovered.
In this article, we will trace weather prediction's journey from ancestral proverbs to modern supercomputer calculations. We will glimpse its forgotten origins when a 6-hour forecast required 6 long weeks. By charting each upward step, we will see how today's expected precision was yesterday's impossible dream. Just as weather forecasting turned whimsy into hard science, the evolution of AI may hold clues to future capabilities still residing in the realm of imagination today.
From Lore to Lightning: The Telegraph's Forecasting Spark
For millennia, we lacked the scientific tools to predict the weather, instead relying on tribal wisdom. If cows huddled together, rain was imminent. A red sky at night promised fair weather tomorrow. Generation after generation memorized these weather lore proverbs.
This began to change in 1835 as Samuel Morse invented the telegraph, allowing weather observations to be shared rapidly across cities for the first time. Now real-time information from upwind regions could inform predictions. While the mathematical models underpinning modern forecasting remained undiscovered, the telegraph marked a pivotal innovation, circulating information at lightning speed.
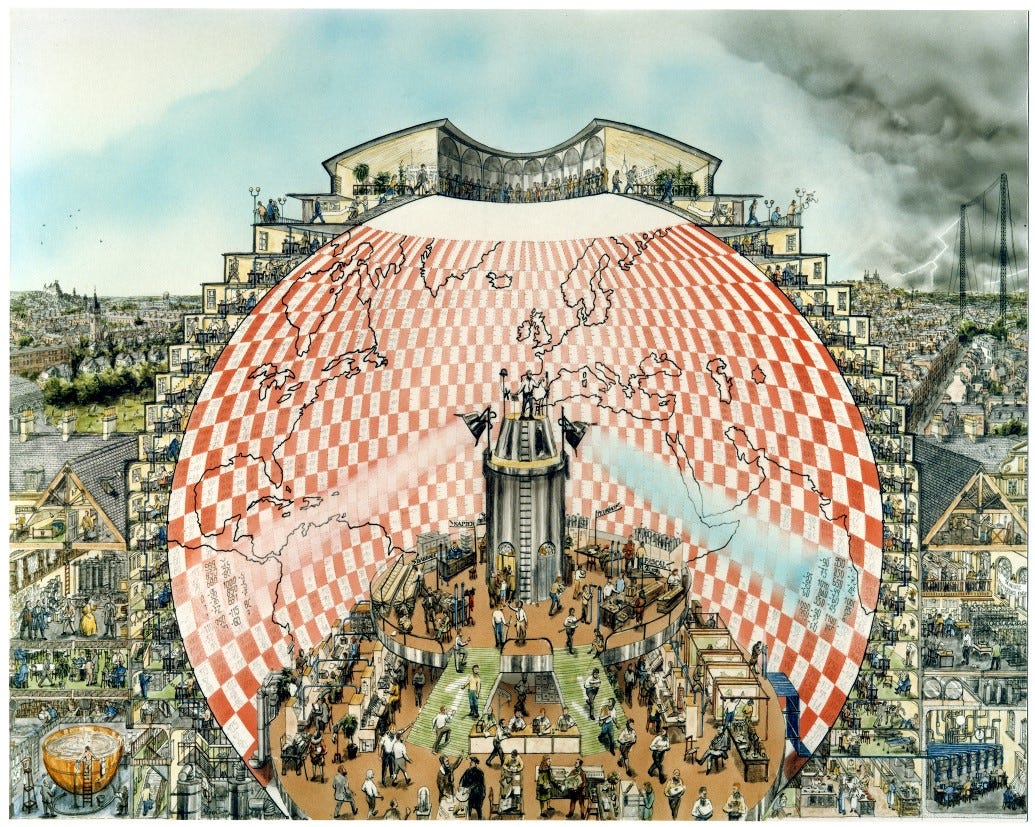
A 6-Week Forecast: Richardson's Impossible Dream
In 1922, Lewis Fry Richardson dreamed the impossible - numerically forecasting the weather. In his groundbreaking book, Weather Prediction by Numerical Process, he outlined a fantastical vision far ahead of its time. Richardson proposed a massive hall filled with thousands of mathematicians. Each would calculate part of the complex differential equations required to model the atmosphere. Runners would ferry the partial solutions between calculation stations to be compiled into a complete forecast.
A myriad computers [people who compute] are at work upon the weather of the part of the map where each sits, but each computer attends only to one equation or part of an equation. The work of each region is coordinated by an official of higher rank. Numerous little "night signs" display the instantaneous values so that neighbouring computers can read them. Each number is thus displayed in three adjacent zones so as to maintain communication to the North and South on the map.
From the floor of the pit a tall pillar rises to half the height of the hall. It carries a large pulpit on its top. In this sits the man in charge of the whole theatre; he is surrounded by several assistants and messengers. One of his duties is to maintain a uniform speed of progress in all parts of the globe. In this respect he is like the conductor of an orchestra in which the instruments are slide-rules and calculating machines. But instead of waving a baton he turns a beam of rosy light upon any region that is running ahead of the rest, and a beam of blue light upon those who are behindhand.
Four senior clerks in the central pulpit are collecting the future weather as fast as it is being computed, and despatching it by pneumatic carrier to a quiet room. There it will be coded and telephoned to the radio transmitting station. Messengers carry piles of used computing forms down to a storehouse in the cellar.
Richardson's description from Weather Prediction by Numerical Process
To demonstrate his methods, Richardson produced by hand a 6-hour weather prediction for two points in central Europe. The feat took at least six weeks of tireless effort. But in 1922, before the invention of modern computers, Richardson's numerical techniques were impractical to execute. The computational power (human or not) required to calculate them was not available.
ENIAC's Computing Dawn: Bringing Forecasts to Life
In the 1950s, the fantastical forecasting vision of Lewis Fry Richardson was finally realized, not by rooms of human computers but by a pioneering machine - the ENIAC. Weighing 30 tons, this early computer marked a new era for numerical weather prediction.
A team of talented scientists programmed ENIAC to generate the first computerized 24-hour weather forecast in 1950. The massive calculation took nearly a full day to complete on ENIAC's room-sized circuits. Led by meteorologists Jule Charney and Philip Thompson, mathematician John von Neumann, and programmer Klara Dan von Neumann, they proved Richardson’s equations could produce predictions given sufficient computing power.
Over the next decades, increasingly powerful computers allowed ever more precise forecasts with faster turnaround times. Technology caught up to imagination, unveiling the next vista in meteorology. ENIAC ushered in modern forecasting.
Vectors to Vistas: Word Embeddings Unlock AI's Potential
Just as early weather forecasting leaned on lore until mathematical models were discovered, today's rapid advancements in natural language AI stand on the shoulders of a pivotal innovation - vector embeddings.
Back in 2013, researchers at Google made a groundbreaking discovery. They found that words could be translated into mathematical vectors representing linguistic patterns detected in massive datasets. Words used in similar contexts clustered closely together in vector space, like birds of a feather flocking together. For instance, "dog" and "bark" often appear together in text, so their vectors are nearer one another.
This breakthrough enabled astonishing new capabilities. Word relationships could now be expressed algebraically, solving analogies like:
king - man + woman = queen
.
Subtracting the "man" vector from "king" and adding "woman" results in a vector very close to "queen." It solves a word puzzle using math!
Later, the transformer architecture leveraged context-based vector generation. For example, "bat" has different meanings in these sentences:
"The bat flew out of the cave."
"The player swung the bat."
Transformers' attention mechanisms can use context words like "flew" or "swung" to produce appropriate "bat" vectors.
Importantly, these techniques apply beyond language, too. Vectors can encode images, audio, time series, and other multimedia data. By extracting patterns from huge datasets, transformers enabled today's multimodal AI capabilities.
Waypoints to the Summit
In weather forecasting, discovering the equations was not the final summit, but a pivotal waypoint enabling future innovation. The differential equations that drove increasingly accurate predictions went on to transform aircraft design, finance, traffic engineering, and more.
Similarly, natural language AI's breakthroughs, like vector embeddings and transformer architectures, are waypoints not summits. The techniques unlocking language's complexity will compound, informing solutions in computer vision, healthcare, science, and other spheres.
Step by step, each discovery brings us closer, revealing new applications once confined to imagination.