CheckMate: AI Will Take the Jobs of Those Using It
A sobering look at how AI's rapid ascent could render human knowledge workers obsolete, taking cues from the era when chess engines checkmated human grandmasters.
In 2023, as debates raged over AI's impending impact, one line sliced through the noise with sobering clarity: "AI won't take your job. It's someone using AI that will take your job." Uttered by Professor Richard Baldwin at the World Economic Forum, this sentiment quickly became a rallying cry for those seeking to calm fears of an AI takeover. Yet, as this article will explore, the true checkmate may lie in AI eventually replacing not just tasks but the very roles of those who wield it as a tool.
Last year, in my article titled "The Centaur Advantage: How the AI-Human Partnership Could Change Us for Better and Worse," I delved into how new technologies have reshaped human advantage. I examined how the advent of GPS reduced barriers to entry for becoming a taxi driver in London's notoriously winding streets. I also traced the origins of "centaur chess" – a game where teams of humans and computers competed against other human-machine hybrids.
However, my exploration of centaur chess stopped in 2005 – the year a seminal event occurred. A team of amateur players armed with three computer programs entered the Freestyle Chess tournament and defeated grandmasters. This served as a definitive data point, proving that those with superior skills in leveraging chess engines could outmaneuver grandmasters ill-equipped to harness technology.
But the story didn't end there. In this article, I aim to pick up that thread and explore what became of centaur chess and, by extension, what it could portend for our jobs in the world of AI.
The Elo Evolution: Mastering the Numbers Game
But before we delve further, we must first understand the Elo rating system – a development that revolutionized how chess players are ranked. The brainchild of Hungarian-American physics professor and chess enthusiast Arpad Elo, it introduced a mathematical approach to player rankings based on game outcomes. Adopted by the World Chess Federation (FIDE) in 1970, Elo ratings ensured each player's score reflected their current skill level, gaining or losing points depending on whether they won or lost against higher- or lower-rated opponents.
When the 1978 ratings were published, American Bobby Fischer topped the list with an Elo score of 2780. As the years progressed, ratings crept higher, with Norway's Magnus Carlsen setting the current human peak of 2882. However, a new contender was emerging – computer chess engines first became competitive in the late 1970s, with Northwest University's program winning the 1977 Minnesota Open. The following year, it reached an Elo score of 2030, still below elite grandmaster levels.
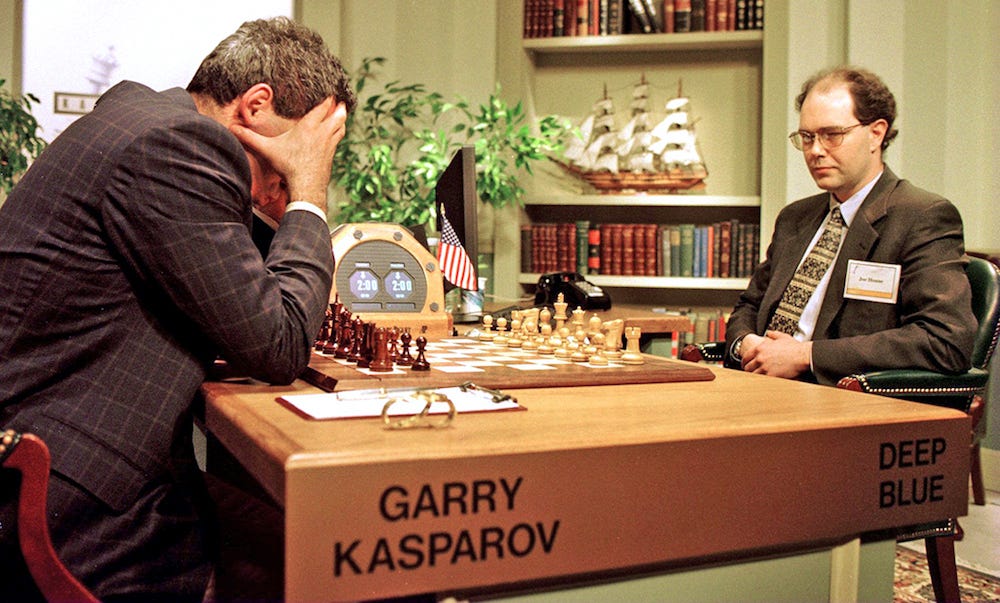
This disparity didn't last. IBM's Deep Thought lost to Garry Kasparov in 1989, but by 1997, Deep Blue had bested the reigning champion and earned an Elo of 2853. Kasparov went on to create Centaur Chess - pitting teams of humans and computers against other hybrid teams.
These "centaur" events continued in various forms until around 2008 when they fell out of favor. One reason: Computers had advanced to an extent where having a human partner provided no advantage. Computer Elo scores continued their inexorable rise, leaving human players behind.
World champion Magnus Carlsen won't play computer chess because "he just loses all the time, and there's nothing more depressing than losing without even being in the game." Today, computers and humans mostly play in their own tournaments.
When Assistants Become Experts: AI's Inexorable Rise
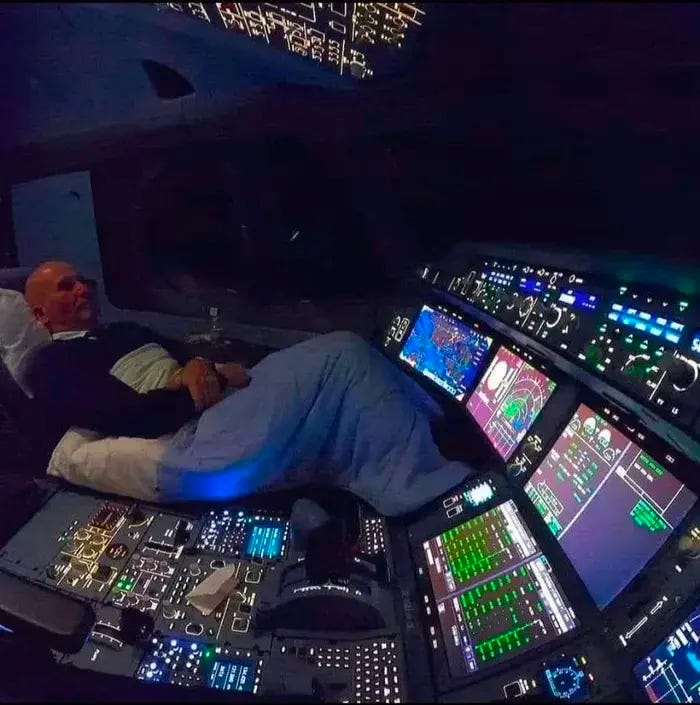
Extrapolating from the experience of the chess world, today's knowledge workers like you and me sit in the late 1990s era. If we assigned Elo ratings for knowledge work capabilities, they've risen from toy programs to approaching human parity - and in some domains, even besting us. Much like the chess grandmasters of that time, our mental model has been to treat AI as an assistive tool, a copilot to leverage. Hence the prevalent notion that "AI won't take your job. It's someone using AI that will take your job."
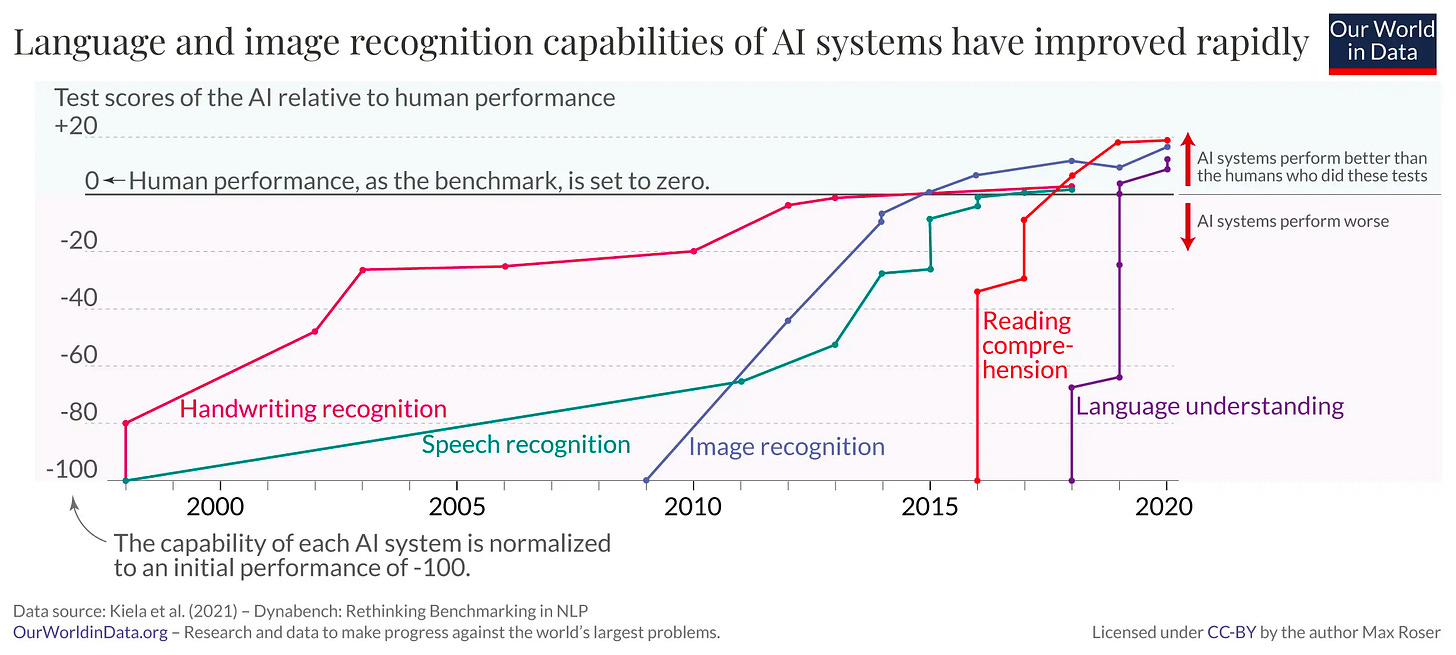
To ground this in a relatable analogy, consider the computer in your doctor's office. Currently, it serves as little more than a digital etch-a-sketch - recording, storing, and retrieving patient information to aid the physician's decision-making. However, models like Google's Med-PALM, which passed the notoriously difficult United States Medical Licensing Examination, portend a shift as AI is integrated into diagnostic assistants. While today the technology augments doctors, there may come a point, like in chess, when these advanced systems surpass human medical expertise altogether.
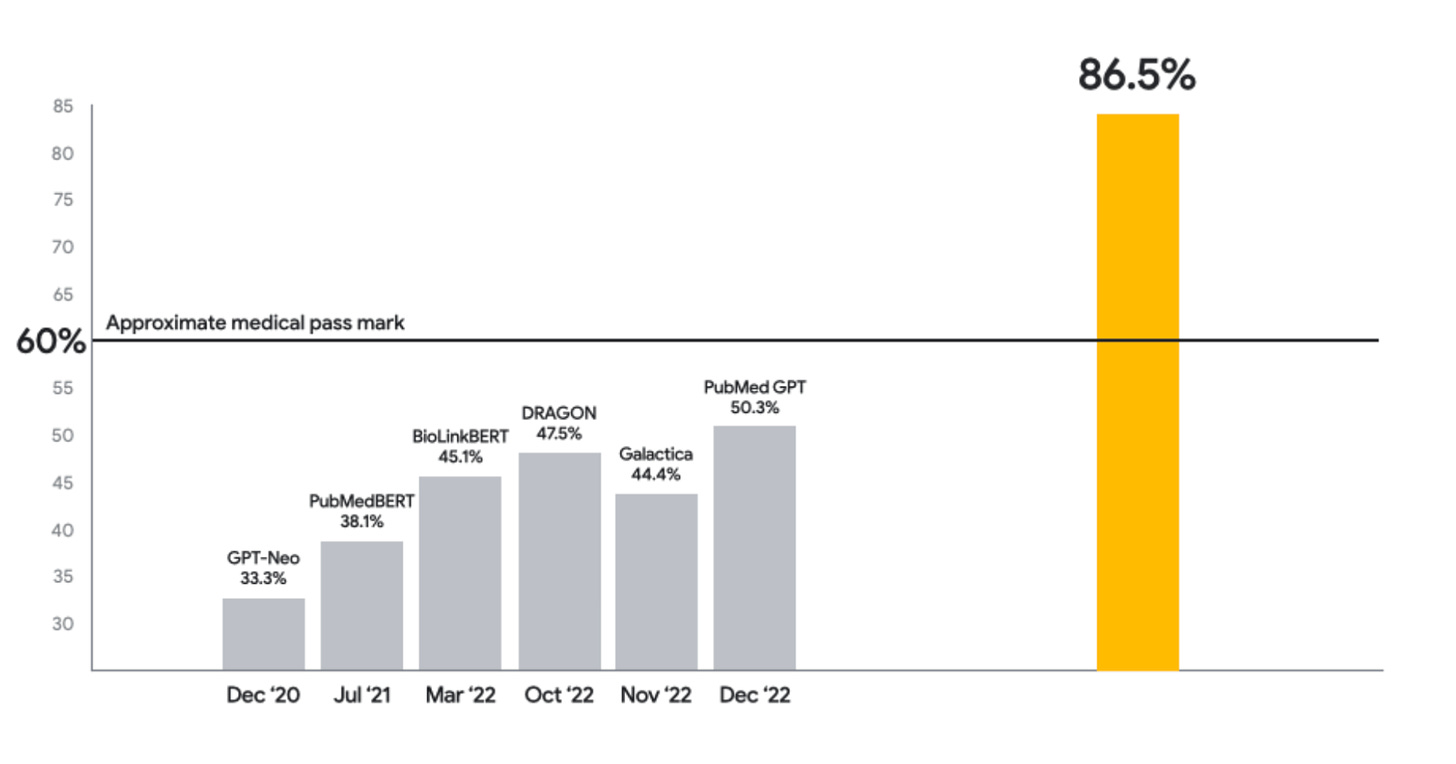
Our initial mental model for adopting AI has mirrored that of the horse-and-buggy driver swapping their horse out for an automobile. We believed workers just needed to learn new skills, like how to operate the new automobile technology, and they would remain productive. However, a better comparison could be that we are actually the horse - and our employer is the driver. In this situation, our boss/customer must decide whether to keep using a human worker/vendor or replace us with an AI automobile.
The twilight of human mastery in chess offers a lesson into a world where computers caught up to human capability, worked with humans, and then left us behind as they rapidly climbed the ladder of ability. This path could serve as an ominous roadmap for how artificial intelligence will progress and ultimately surpass human workers in various domains. What began as a collaborative era with "centaur" human-machine partnerships may prove to be a mere transition period before AI renders conventional knowledge workers obsolete.